AI for Battery
From Machine Learning to Deep Learning

Our research group is pioneering the application of artificial intelligence (AI) in battery research, utilizing both machine learning and deep learning techniques to tackle complex challenges in the field. By integrating AI technologies into battery research, our group aims to accelerate the development of advanced energy storage solutions. From enhancing imaging techniques to forecasting the charge/discharge curves and accurately detecting the degradation mechanisms , we are committed to pushing the boundaries of how machine learning and deep learning can contribute to the future of battery technology.
Related publications :
-
Artificial neural network approach for multiphase segmentation of battery electrode nano-CT images
-
Self-supervised image quality assessment for X-ray tomographic images of Li-ion battery
-
Artificial intelligence applied to battery research: hype or reality?
-
Denoising of 4D-STEM Dataset using Pix2Pix GAN and Artifact Reduction
Battery Characterization
X-ray Nano Computed Tomography & Electrochemical in situ Cell

Our research group focuses on advancing the 3D local characterization of battery materials using X-ray nano computed tomography (nano-CT) techniques. We aim to understand the microstructural properties of battery electrodes at the nanoscale and how these properties influence overall battery performance. This integration (Zernike Phase Contrast, Holography, XANES spectroscopy) provides a comprehensive view of both the structural and compositional changes occurring within the battery during use. Our overarching goal is to enhance the understanding of battery material behavior through these cutting-edge 3D imaging techniques. By doing so, we contribute to the development of more efficient, durable, and higher-performing energy storage systems.
Related publications :
-
3D Quantification of µ-structural Properties of NMC532 by X‐Ray Holographic Nano‐Tomography
- X-ray Nano-CT in Zernike Phase Contrast for Studying 3D Morphology of Li–O2 Battery Electrode
- Towards a Local In situ X‐ray Nano-CT under Realistic Cycling Conditions for Battery Research
- Combining X-ray Nano-CT and XANES for 3D Operando Monitoring of Li Spatial Composition evolution in NMC
Battery Characterization
4D-STEM structural mapping
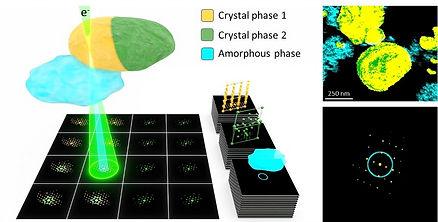
Our research group is dedicated to advancing the 4D-Scanning Transmission Electron Microscopy (4D-STEM) Automated Crystallographic Orientation Mapping (ACOM) technique for local structural characterization of battery materials. We have developed improved pattern matching methods in 4D-STEM ACOM by implementing adaptive sub-pixel peak detection and image reconstruction, enhancing the precision of crystallographic analyses. This approach allowed us to correlate structural and chemical information, providing comprehensive insights into battery material behaviors. Collectively, our work contributes to the development of more efficient and durable energy storage systems by enhancing the understanding of battery materials at the atomic level.
Related publications :
Battery Characterization
Electrochemical in situ TEM
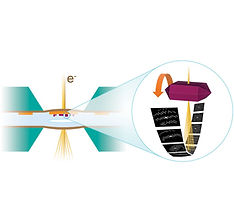
Our research group focuses on advancing liquid electrochemical transmission electron microscopy (TEM) techniques to monitor reactions at the nanoscale in real-time within battery materials. This technique allows for detailed analysis of crystallographic transformations as they occur. Our studies provided valuable information on the material's electrochemical performance and structural stability at the nanoscale, contributing to the development of high-performance micro-energy storage devices. Furthermore, by coupling liquid electrochemical TEM with mass spectrometry, we have explored the reactions occurring in battery . Through these innovative methodologies, our group aims to elucidate fundamental processes in battery operation and failure, ultimately contributing to the design of more efficient and durable energy storage systems.
Related publications :